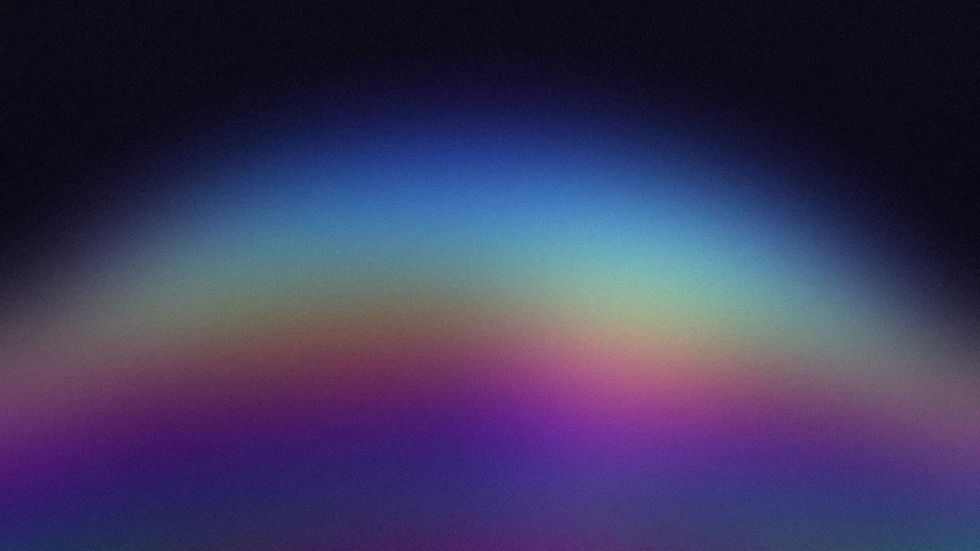

Matchimus
Based on our operational experience with reconciliations in the banking industry our team set out to develop the industry's most effective and accurate transaction matching system. With this goal, Matchimus was designed from the ground up for this specific business purpose.
The Reconciliation Matching Problem
Financial transaction data often suffer from quality issues and even with carefully configured rules can’t be reliably matched, leaving your reconciliation team to manually match thousands or even millions of transactions. Not only is this exhausting but your downstream processes have to wait for your teams to complete all this work before the reconciliations can be closed out.
Additionally, rules engine and user misjudgments sometimes lead to costly mismatches that may only be discovered much later in the overall operations workflow, requiring corrective actions in multiple downstream processes and systems.
The Matchimus Solution
Matchimus machine learning algorithms have been purpose-built specifically for transactional data matching taking into account the operational characteristics of the matching process. This includes automatically keeping each model up to date to handle ongoing changes in the operational data.
Matchimus optimization performs a comprehensive 'what-if' on all possible matchings, enabling it to zero-in on the best matches and crystalize its decisions leading to groundbreaking accuracy, even when operating with poor transaction data quality.
With its advanced match intelligence using specifically honed machine learning algorithms, Matchimus dramatically reduces your manual matching workload, letting your team complete reconciliations faster and with less effort. In case-studies Matchimus typically delivers a 60-90% reduction in manual matching when compared to the market-leading rules engines. Even our competitors call our technology disruptive.
In addition to high match rates, Matchimus maintains a greater than 99.9% accuracy which virtually eliminates mismatches, keeping your recs and post-rec processes ship-shape.
Beyond the Limitations of Rules
Rules based matching works well when your data quality is great but when data quality is not so good, your rules engine can leave thousands of transactions for your team to manually match, draining their energy and focus and creating delays in your daily reconciliation and downstream processes.
Matchimus goes beyond rules based matching by combining the power of machine learning with match possibilities consideration, delivering high match rates and pinpoint accuracy even when transaction data quality is poor.
Rules Limitation : Attribute significance
Problem
Rules use attribute conditions to decide which transactions should be matched together (for example amount or date). With rules based matching, the importance of each attribute in a rule is encoded in the form of the rules ordering. For example a rule with an exact amount condition and a date condition with a tolerance of 1 day may be given a higher priority than a rule which has an amount tolerance of $10 and exact date match. This represents the fact that exact amount condition is more significant for matching than an exact date condition. However, a rule ordering cannot accurately represent an exact numerical value for the true significance of this attribute condition. Additionally, the rule structure and ordering must be defined by the user when setting up the rules, and is therefore subject to adhoc construction.
Solution
Matchimus addresses this problem by accurately representing attribute significance as a numerical value. Additionally it determines these values using principled statistical analysis from the training data, ensuring their accuracy.
Rules Liability : Proliferation
Problem
For more complex reconciliations, many rules may be needed to handle the data characteristics. For example, for a multi currency cash rec there may need to be a separate rule for each currency, date condition and expense type, leading to a 'combinatorial explosion' in the number of rules which need to be created.
Solution
Matchimus automatically learns characteristics such as currency specific tolerances, removing the need for these to be defined by the user. Additionally the combination of different attributes is handled automatically without the need for explicit combinations to be defined by the user.
Rules Liability : Maintenance
Problem
Data characteristics can vary over time and this requires match rules to be periodically reviewed, modified and re-validated on an continual, ongoing basis.
Solution
With Matchimus, this continual manual tweaking is no longer needed as adaptation to changes in data is automatically ensured with regular automated training updates
Rules Limitation : Shortsighted Decision making
Problem
The application of a set of prioritized matching rules is intrinsically a 'greedy' approach whereby the current match rule being applied will match two unmatched transactions as long they meet the current rule condition. This does not take into account any other viable matches for these transactions which might be implied by other, lower priority rules. This shortsightedness can lead to erroneous matches as these alternative, lower priority viable matches are sometimes the actual correct match.
Solution
Matchimus avoids this problem by performing a comprehensive 'what if' analysis of all possible match decisions for a given set of transactions, using that to accurately weigh the pros and cons of each match, taking into account all alternative possibilities for every involved transaction.
Rules Limitation : Accuracy
Problem
How do you know if a rule will lead to accurate results? For strict match rules which require a unique common reference present on both transactions it can be said that such a match is 100% accurate. But what about rules which do not require a unique common reference? How can this be answered? Trial and error is the usual approach, set up a rule run the matching and check if all of its created matches are correct. The more 'fuzzy' the rule, the more the risk of erroneous match creation so this can require multiple iterations.
Solution
Matchimus answers this problem by using learned Bayesian statistics to calculate an accurate probability for each possible match and then refines these probabilities further by taking into account all possible matches for each transaction. This allows the use of high accuracy thresholds of 99.9% accuracy and even with this strict threshold still provides match rates which goes beyond rules.
Get a Demo of Operartis
01
Want to get started? Fill in the form to start the conversation about how Operartis can help your business.
02
Want to see proof of value? We'll measure the match rate improvement on your chosen reconciliation data and provide an automation report detailing your ROI before you commit to purchase.
:+1-212-810-0264