In the fast-paced retail industry, efficiency is paramount. For a major retailer, the daily expense reconciliation process was a significant drain on resources, despite utilizing a leading cloud-based reconciliation engine.
While achieving a respectable 93% match rate through 13 custom-crafted rules, the remaining 7% translated to thousands of transactions requiring manual intervention.
This case study explores how Operartis' Matchimus, powered by machine learning for matching, transformed their reconciliation process, preventing a costly relocation and significantly boosting efficiency.
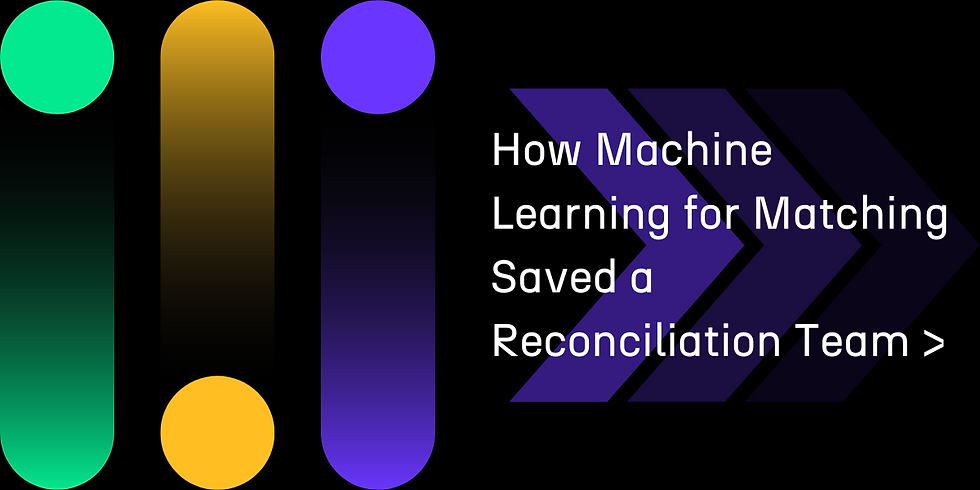
The Challenge: Manual Matching and Cost Pressures
The retailer faced a common challenge: balancing automation with the need for manual intervention. Their existing cloud-based reconciliation engine, while effective, still left a substantial volume of transactions requiring manual matching. This manual effort was not only time-consuming and expensive but also created operational bottlenecks.
As highlighted on our reconciliation automation page, "manual reconciliation is prone to errors and delays." The retailer was experiencing this firsthand, with a significant amount of manual work leading to inefficiencies and increased operational costs. In an effort to mitigate these costs, the retailer was considering relocating their reconciliation team to a lower-cost location.
The Solution: Matchimus and the Power of Machine Learning for Matching
Recognizing the limitations of their rules-based system, the retailer sought a more intelligent and efficient solution. They turned to Operartis and their Matchimus platform, which leverages machine learning for matching to automate complex reconciliation processes.
The implementation was remarkably swift and straightforward. Following a single training run using the retailer's historical match data, Matchimus was able to learn the patterns and nuances of their transactions. This demonstrates the power of machine learning for matching to quickly adapt and optimize reconciliation processes.
The Results: A Dramatic Improvement in Efficiency and Accuracy
The results were nothing short of transformative. Matchimus increased the customer matching rate from 93% to an impressive 98%. This 5% increase translated to an 80% reduction in manual matching work. The retailer's reconciliation team was freed from the tedious task of manually processing thousands of transactions, allowing them to focus on more strategic, value-added activities.
Furthermore, Matchimus achieved a 95% reduction in customer mismatches. This dramatic improvement in accuracy significantly reduced operational risk and improved the overall integrity of the retailer’s financial data. As Operartis emphasizes, "AI can identify and resolve discrepancies with greater accuracy than manual processes."
Key Statistics:
Initial Auto-Match Rate: 93% (using a rules-based system)
Matchimus Auto-Match Rate: 98%
Reduction in Manual Matching: 80%
Reduction in Mismatches: 95%
The Impact: Cost Savings, Efficiency, and Team Retention
The implementation of Matchimus delivered significant benefits to the retailer:
Cost Savings: The dramatic reduction in manual matching and mismatches resulted in substantial cost savings, eliminating the need for a costly relocation.
Increased Efficiency: The automated reconciliation process streamlined operations, freeing up valuable resources and improving productivity.
Improved Accuracy: The significant reduction in mismatches enhanced data integrity and reduced operational risk.
Team Retention: Preventing the team relocation maintained valuable expertise and team morale.
Machine Learning for Matching: The Future of Retail Reconciliation
This case study demonstrates the transformative potential of machine learning for matching in the retail industry. By automating complex processes like daily expense reconciliation, retailers can achieve significant improvements in efficiency, accuracy, and cost savings.
As retailers continue to face increasing pressure to optimize operations and reduce costs, machine learning will play an increasingly vital role in driving innovation and operational excellence. Operartis' Matchimus platform is a testament to the power of machine learning for matching to revolutionize reconciliation and empower retailers to achieve their strategic objectives.
By adopting AI-powered solutions, retailers can not only improve their bottom line but also enhance their ability to adapt to the ever-changing retail landscape. Machine learning for matching is not just a technological advancement; it's a strategic imperative for retailers seeking to thrive in a competitive market.
What's Next?
Don't settle for the limitations of manual reconciliations. Operartis offers a glimpse into the future of financial services with machine learning in the retail industry – a future powered by data-driven insights and AI.
Let us help you transform reconciliations from a burden into a strategic advantage.
Get a quote personalized to your use case with our Proof of Value (PoV) assessment. We'll measure the match rate improvement on your reconciliation data and provide an automation report detailing your ROI before you commit to purchase.
Let's talk it over – schedule your demo to increase efficiency, improve exception management, reduce costs, and enhance visibility into your financial data.